9/9/2021 Jenna Kurtzweil
Written by Jenna Kurtzweil
Researchers at the Beckman Institute for Advanced Science and Technology combined label-free microscopic imaging with artificial intelligence to quickly detect and classify SARS-CoV-2. Beckman researchers paired microscopy with artificial intelligence to develop a COVID-19 test that’s fast, accurate, and cost-effective. All we need to do is say “ahh.” Many of us have encountered or experienced a COVID-19 test. Like the pandemic itself, frequent screening has become part of daily life. As SARS-CoV-2 continues to be a formidable foe, our strategies to detect and classify the virus must remain agile and sophisticated.
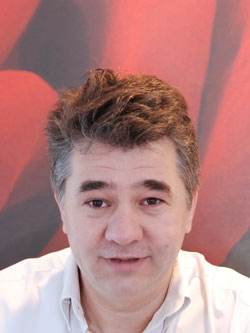
Enter Beckman researcher Gabriel Popescu, a UIUC professor of electrical and computer engineering, and his interdisciplinary team. Their study, “Label-free SARS-CoV-2 detection and classification using phase imaging with computational specificity,” was published in Light: Science and Applications-Nature.
Beginning in May of 2020 and coming to fruition amid a global crisis, the project’s timeline runs parallel to the pandemic that prompted it. Characteristic of a Beckman team, the researchers’ first step was identifying an opportunity to innovate; they observed that while many techniques currently exist to test for SARS-CoV-2, none use a label-free optical approach.
The minuscule size of a single particle makes relying on sight alone a near impossibility, even with a microscope. Electron microscopy is useful for imaging a particle’s structure, but extensive preparation is required to ensure a sample’s visibility. Though necessary, this process can obscure the desired image. Popescu’s team turned to a technique developed at Beckman typically reserved for visualizing cells: spatial light image microscopy, which facilitates chemical-free (or label-free) imaging.
“An electron microscope provides a clear image, but it requires extensive sample preparation,” said Neha Goswami, a graduate student in bioengineering and a 2021 recipient of Beckman Institute’s Nadine Barrie Smith Memorial Fellowship. “Applying SLIM for virus imaging is like looking at something without your glasses on. The image is blurry due to the viruses being smaller than the diffraction limit. However, owing to the high sensitivity of SLIM, we can not only detect the viruses, but also differentiate between different types.” Fortunately, the researchers identified a creative way to identify the viruses based on SLIM data: artificial intelligence. With the right training, an advanced deep neural network can be programmed to recognize even the blurriest of images.
The AI discerned between four particles: SARS-CoV-2, H1N1, HAdV, and ZIKV. The preclinical trial resulted in a 96% success rate for SARS-CoV-2 detection and classification. They introduced the AI program to a pair of images: a stained SARS-CoV-2 particle producing fluorescence, and a phase image captured with a fluorescence-SLIM multimodal microscope. The AI is trained to recognize these images as one and the same. Easily recognizable, the fluorescence-stained image functions like training wheels; with enough repetition, the machine learns to detect the viruses directly from the SLIM, label-free images without the added support. After detection comes differentiation: discerning SARS-CoV-2 from other types of viruses and particles.
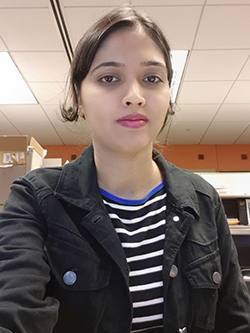
“We made life tough on the machine,” Goswami said. “We gave it dust, beads, and other viruses to train and learn to pick the virus out of a crowd as opposed to identifying when it is by itself.”
The AI learned to discern between SARS-CoV-2 and other viral pathogens such as H1N1, or influenza A; HAdV, or adenovirus; and ZIKV, or Zika virus. The preclinical trial was highly successful, resulting in a 96% success rate for SARS-CoV-2 detection and classification. “This notable success is due to our team of experts from several different disciplines who came together with a unique goal: to create the fastest, most affordable, and scalable test possible. Our current efforts are focused on demonstrating this approach in the clinic and deploying it worldwide for COVID and potentially other infectious diseases,” Popescu said. The project’s goal is a sensitive and specific viral breath test detection system that aids in viral diagnostics and in transmission prevention strategies; today, this could take the form of a rapid, high-throughput, low-cost COVID-19 test with the potential for portability and point-of-care action.
With clinical validation pending, researchers speculate that a COVID-19 test conducted with this method would look something like this: the subject would wear a face shield, onto which a clear glass slide would be attached; they would then complete an activity wherein their breath becomes fixed to the slide (like reading a paragraph out loud). The slide, and any particles attached to it, would be imaged and analyzed to detect any viruses present. “There are two key advantages to this kind of COVID test,” Goswami said.
“The first is speed: the duration can be of the order of one minute. The second is that we are not adding any chemicals or modifications to the samples provided. All we’d be paying for is the cost of the face shield and the slide itself.” From a clinical perspective, the impact of such innovative diagnosing capabilities is pronounced.
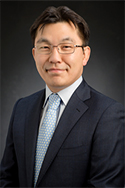
"Early intervention via rapid diagnosis of COVID-19, combined with contact tracing, will significantly reduce COVID-19 transmission, morbidity, and mortality," said Nahed Ismail, a professor of pathology and medical director of the Clinical Microbiology Lab at the University of Illinois at Chicago. This highly adaptive AI programming could help address future pandemics, not just COVID-19.
“We need fast detection of diseases,” Goswami said. “Not only COVID, but others. We can and should put our efforts together, both in terms of optics and AI, to try and find out just how far we can go.” This cutting-edge research is a result of collaboration between scientists from the Beckman Institute, the University of Illinois Urbana-Champaign, and the University of Illinois at Chicago. “The amazing thing about this project is that we can bring lab work to clinical trials in a very short time,” said Helen Nguyen, the Ivan Racheff Professor in Civil and Environmental Engineering at UIUC and project collaborator.
Other collaborators included: Rashid Bashir, dean of the Grainger College of Engineering at UIUC; Hyun J. Kong, a professor of chemical and biomolecular engineering at UIUC; and Catherine Best-Popescu, a research assistant professor of bioengineering at UIUC.
Editor’s note: the paper associated with this work can be found at https://rdcu.be/cwtLq.